Working with national insurers and PBMs, Certilytics deploys its sophisticated predictive analytics in support of value-based care management programs at dozens of large, self-funded employers.
A recent analysis of those programs showed they save participating employers an average of over $200 per member, per year (PMPY), relative to previous care-management strategies. For a typical employer—with about 30,000 participating beneficiaries—this translates to annual savings of more than $6 million.
To achieve these savings, we help our customers move from retrospective reporting to prospective predictive analytics—identifying members for outreach before they’ve incurred significant costs.
This includes identifying members at risk for high-cost events, such as emergency room visits, along with members at risk for developing chronic diseases, such as diabetes. In the case of emerging clinical risk, we group members into risk buckets based on their likelihood of developing chronic diseases, enabling our clients to track progress in using care management strategies to move members from high risk to medium risk, or medium risk to low risk. We also predict a member’s likelihood of engagement, maximizing the chances that clinical interventions will be effective.
Our advanced, predictive approach is not intended to replace an employer’s existing health benefit and care management programs. Instead, we seek to optimize those programs by acting as a centralized data warehouse hub that can be deployed with ease and integrates with existing resources. We help employers maximize the potential of their care management investments by analyzing utilization and engagement data to predict which beneficiaries will take advantage when directed to the services that will have the greatest impact on their health.
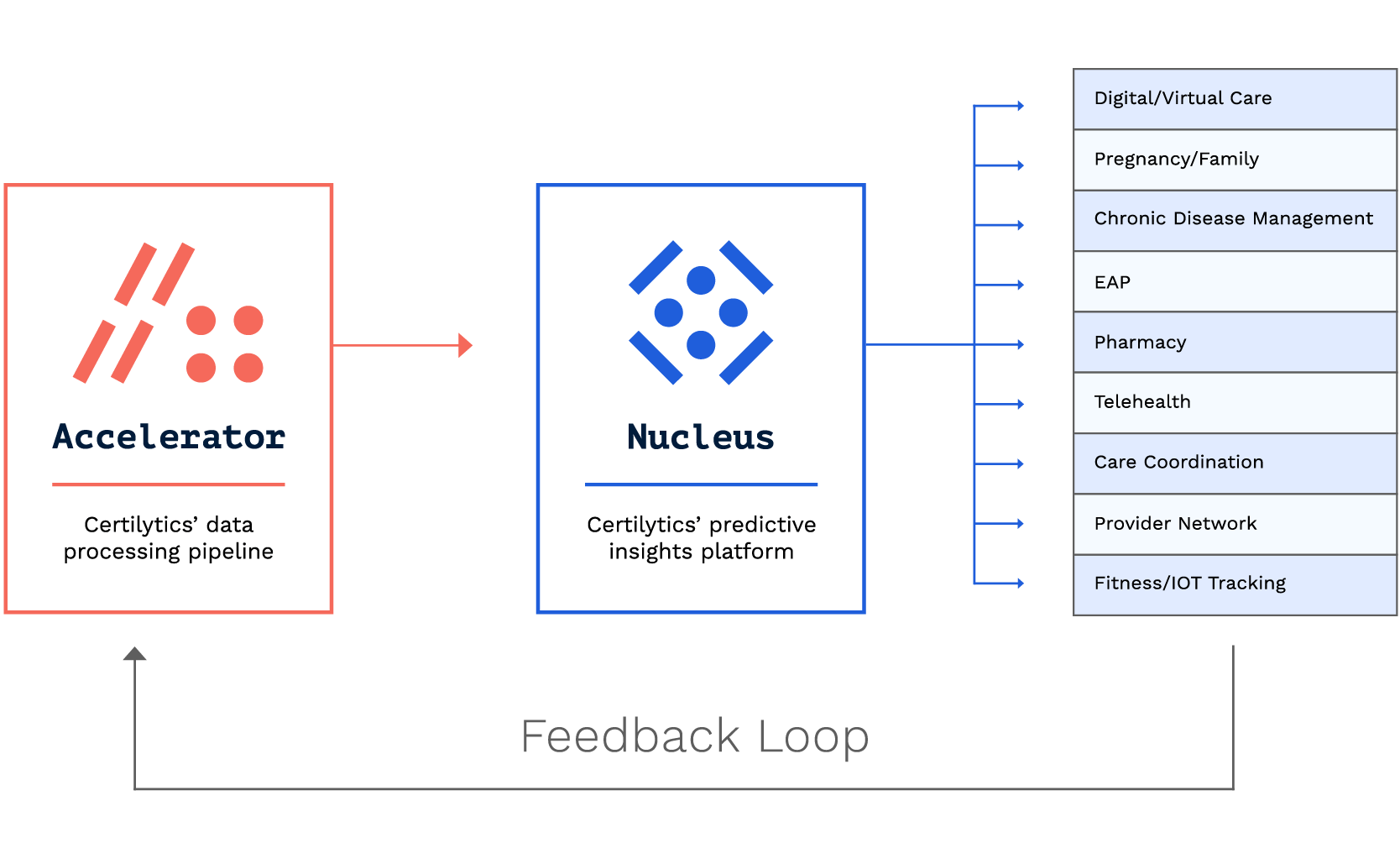
The data from our analysis shows our sophisticated approach to value-based care management is having the intended effect, with cost savings that correspond with a positive shift in utilization patterns, including increased medication adherence.
“As the predictive analytics space becomes more crowded, modelers must move beyond quoting validation accuracies and show real-world results,” said Certilytics Chief Data Scientist Robert Dwyer, PhD. “In this analysis we used best-practice causal modeling approaches to show that our analytics-driven programs actually achieve real-world savings on the order of hundreds of dollars PMPY.”
The Problem with Traditional Approaches to Care Management
Traditional approaches to value-based care management typically include identifying members for intervention based on retrospective factors such as:
- Open Gaps in Care
- Historic Utilization / Costs
- Financial Risk
Unfortunately, these factors fail to provide care managers with a complete picture of their members’ emerging clinical risk profile. By the time care managers react based on open gaps in care or avoidable utilization, members have oftentimes already incurred a significant (and avoidable) spike in their healthcare costs.
The Certilytics Approach
Certilytics enhances the member identification process with prospective analytics that account for a member’s likelihood of modifying behavior if targeted by a care manager.
Rather than focusing on members already diagnosed with chronic conditions, Certilytics’ predictive models identify members at high risk of developing chronic conditions, enabling earlier interventions to prevent high-cost events. These models are aggregated into the Certilytics Health Index (CHI), an AI-powered proprietary algorithm that stratifies members based on the optimal combination of three factors:
- Emerging Clinical Risk: the prospective likelihood of a member developing chronic diseases or utilizing high-cost services such as the Emergency Room
- Prospective Financial Risk: a member’s predicted financial risk over the next twelve months based on medical history, utilization patterns, gaps in care, demographic data, and other non-traditional data sources
- Propensity for Engagement: the likelihood a member will adjust behaviors because of outreach from a nurse or care manager
Because Certilytics’ models account for a member’s propensity for engagement, Certilytics has a substantial edge over traditional approaches to value-based care management.
Contact us to hear more about our recent analysis and how we can save your organization millions of dollars per year through value-based care management.